Imagine if machines could read our minds. How would they understand our emotions, interpret our thoughts, and gauge our sentiments? While sentiment analysis may sound like a form of mind-reading, it’s actually a fascinating task performed by AI models. In this article, we’ll explore the question: Is sentiment analysis a mind-reading task for models? Let’s uncover the truth behind this intriguing concept.
Sentiment analysis, also known as opinion mining, is the process of analyzing and determining the sentiment expressed in a piece of text. It involves deciphering whether the text conveys a positive, negative, or neutral sentiment. But here’s the catch – sentiment analysis doesn’t rely on mind-reading abilities. Instead, it leverages advanced algorithms and machine learning techniques to understand the emotions and opinions expressed by individuals.
So how do these models accomplish sentiment analysis? Well, they use a combination of natural language processing (NLP) and machine learning algorithms to analyze the sentiment expressed in text data. Through the analysis of language patterns, context, and linguistic cues, these models can discern the emotions and opinions behind written text. It’s an impressive feat, but it’s important to remember that sentiment analysis is an algorithmic process, not a form of mind-reading.
In conclusion, sentiment analysis is an incredibly powerful tool that allows machines to understand human emotions and opinions expressed in text. Despite its seemingly mind-reading capabilities, it’s important to recognize that sentiment analysis relies on algorithms and machine learning techniques, rather than reading our minds directly. So the next time you come across sentiment analysis, remember that it’s a remarkable application of AI and data analysis, but still far from the realm of mind-reading. Let’s dive deeper into the fascinating world of sentiment analysis and unravel its inner workings!
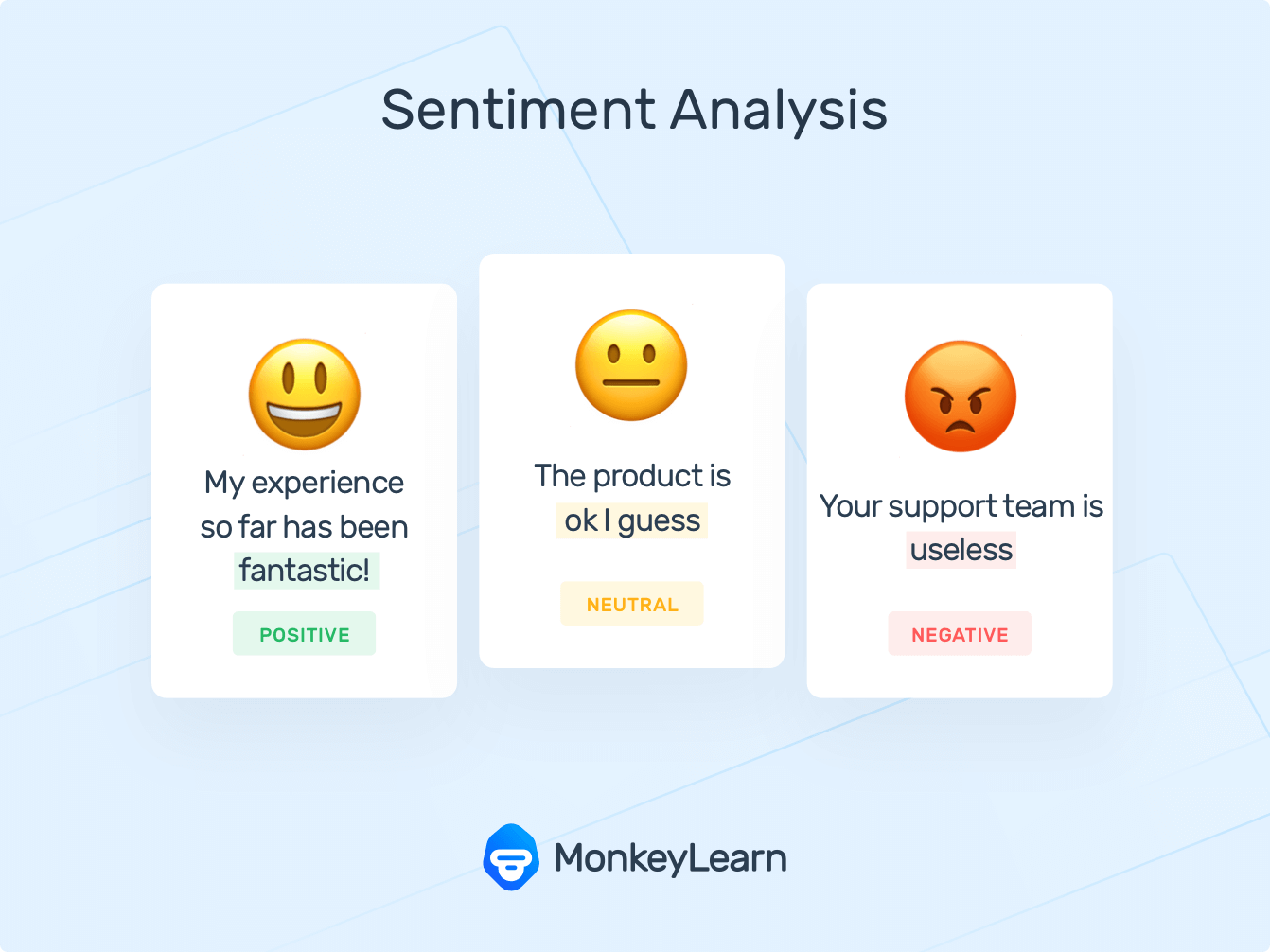
Contents
Is Sentiment Analysis a Mind-Reading Task for Models?
When it comes to understanding human emotions, sentiment analysis is a powerful tool. But is it accurate enough to be considered a mind-reading task for models? Sentiment analysis, also known as opinion mining, is the process of analyzing text data to determine the sentiment or emotional tone expressed within it. It has gained significant popularity in recent years, as businesses and researchers strive to understand and utilize the vast amounts of textual data available on the internet.
How Does Sentiment Analysis Work?
Sentiment analysis involves utilizing natural language processing (NLP) techniques to train models to recognize and interpret emotions conveyed in text. These models are trained on extensive labeled datasets, where human annotators assign sentiment labels to snippets of text. The models learn to identify patterns and linguistic cues that are indicative of positive, negative, or neutral sentiment.
The most common approach to sentiment analysis is using a machine learning algorithm, often based on deep learning methods such as recurrent neural networks (RNNs) or transformers. These models are highly effective at processing and understanding large volumes of text data, enabling them to provide accurate and reliable sentiment analysis on a vast scale.
While sentiment analysis models are capable of identifying emotional signals, it is crucial to understand that they are not actually reading minds. Instead, they are trained to recognize patterns and associations between specific textual elements and corresponding sentiments. They rely on the textual context and underlying linguistic cues rather than directly accessing the writer’s thoughts or intentions.
The Benefits of Sentiment Analysis
Sentiment analysis offers numerous benefits across various industries. In the business world, understanding customer sentiment is crucial for market research, brand management, and customer relationship management. By analyzing social media posts, customer reviews, and feedback surveys, businesses can gain valuable insights into customer satisfaction, brand perception, and product performance.
Furthermore, sentiment analysis plays a vital role in reputation management and crisis response. By monitoring sentiment in real-time, organizations can identify and address negative sentiment before it escalates into a full-blown crisis. This enables businesses to manage their online reputation effectively and maintain a positive brand image.
Additionally, sentiment analysis has applications in political analysis, public opinion tracking, and even healthcare. It allows governments and policymakers to gauge public sentiment on various issues, helping them make informed decisions and understand societal attitudes. In healthcare, sentiment analysis can be utilized to monitor patient feedback and sentiment towards healthcare providers, facilitating improvements in patient experiences.
Sentiment Analysis vs. Mind-Reading: Understanding the Limitations
While sentiment analysis is a valuable tool, it is important to recognize its limitations. Sentiment analysis models can sometimes struggle with context-dependent sentiment. For example, the phrase “I’m dying of laughter” may be interpreted as negative sentiment by a model that lacks the ability to recognize idiomatic expressions or sarcasm.
Furthermore, sentiment analysis models are trained on large, diverse datasets, often containing biases and stereotypes. As a result, they can inadvertently propagate and amplify these biases in their analysis. It is crucial to employ techniques such as data augmentation and bias detection to mitigate these issues and ensure fair and unbiased sentiment analysis.
In conclusion, sentiment analysis is a powerful tool for understanding and extracting sentiment from text data. While it is not a mind-reading task for models, sentiment analysis can provide valuable insights into human emotions and opinions. By leveraging sentiment analysis effectively, businesses and researchers can make informed decisions, improve products and services, and better understand their audience.
The Future of Sentiment Analysis
The future of sentiment analysis looks promising, with advancements in areas such as deep learning, contextual understanding, and sentiment-specific models. Researchers are continually striving to improve the accuracy and interpretability of sentiment analysis models.
Contextual Understanding: Beyond Simple Sentiment
One of the key challenges in sentiment analysis is capturing the nuanced meanings and context-dependent nature of sentiment. Researchers are exploring methods to incorporate contextual understanding into sentiment analysis models. This involves considering the broader context in which the text is written, including the author’s background, cultural factors, and the overall narrative.
By incorporating contextual understanding, sentiment analysis models can provide more accurate and nuanced analyses, avoiding misinterpretations and capturing the subtle nuances of sentiment expressed in text.
Sentiment-Specific Models: Focusing on Domain Expertise
Another promising avenue in sentiment analysis is the development of sentiment-specific models that focus on specific domains or industries. These models are trained on domain-specific data and can provide more precise sentiment analysis tailored to specific contexts.
For example, sentiment analysis models trained specifically on customer reviews in the hospitality industry can provide more accurate and domain-relevant sentiment analysis for businesses in that domain. This shift towards domain-specific models allows for more targeted and reliable sentiment analysis in niche industries.
Emotion Analysis: Going Beyond Positive and Negative
Traditional sentiment analysis models primarily focus on binary sentiment classification, categorizing text as either positive, negative, or neutral. However, emotions are more nuanced and multidimensional. Researchers are exploring methods to incorporate emotion analysis into sentiment analysis models.
This involves expanding the classification spectrum to include a wider range of emotions, allowing for a more comprehensive understanding of sentiment. By incorporating emotion analysis, sentiment analysis models can capture complex emotional nuances, providing deeper insights into the emotional landscape of individuals and communities.
In conclusion, the future of sentiment analysis holds exciting possibilities. Researchers are continuously pushing the boundaries of sentiment analysis to capture context, enhance domain-specific analysis, and incorporate emotion analysis. These advancements will enable more accurate and nuanced sentiment analysis, empowering businesses, researchers, and decision-makers to gain valuable insights from textual data with greater precision and depth.
Key Takeaways: Is Sentiment Analysis a Mind-Reading Task for Models?
- Sentiment analysis is a process where models try to understand the emotions expressed in a piece of text.
- Models analyze words, phrases, and context to determine if the sentiment is positive, negative, or neutral.
- However, sentiment analysis is not mind-reading as it cannot decipher thoughts or intentions behind the text.
- Models rely on patterns and pre-trained data to make predictions about sentiment.
- While useful, sentiment analysis has limitations and may not always accurately reflect human emotions.
Frequently Asked Questions
Welcome to our FAQ section where we answer some common questions about sentiment analysis and the capabilities of AI models. Explore the fascinating world of sentiment analysis and learn how it works!
What is sentiment analysis?
Sentiment analysis is a technique used to determine the sentiment or emotion expressed in text. It involves analyzing words, phrases, and context to classify the sentiment as positive, negative, or neutral. It is a vital tool that helps businesses understand public opinion, customer feedback, and social media trends.
AI models are trained to recognize patterns and language cues that indicate sentiment in text. By using natural language processing algorithms, sentiment analysis algorithms can accurately assess text sentiment, enabling businesses to make informed decisions and tailor their strategies accordingly.
How do AI models perform sentiment analysis?
AI models perform sentiment analysis by utilizing machine learning algorithms. These algorithms learn from large datasets of labeled text, where humans have already labeled each text as positive, negative, or neutral. The models analyze millions of data points, taking into account language patterns, phrases, and contextual information.
Using this training data, AI models develop a deep understanding of sentiment. When presented with new text, the models apply the knowledge gained during training to classify sentiment accurately. It’s important to note that while AI models can analyze sentiment, they are not capable of mind-reading or understanding emotions the way humans do.
Can AI models accurately predict sentiment?
AI models have shown remarkable accuracy in predicting sentiment, often surpassing human performance. However, it’s important to note that sentiment analysis is a challenging task due to the complexities of human language and context. While AI models can achieve high accuracy, there are still cases where the analysis may not align exactly with human perception.
AI models are constantly improving through ongoing training and refinement, but they are not infallible. Human review and human expertise play a crucial role in validating and fine-tuning sentiment analysis results to ensure better accuracy and minimize any potential biases that may be present.
What are the practical applications of sentiment analysis?
Sentiment analysis has numerous practical applications across various industries. It helps businesses understand customer sentiment towards their products or services, allowing them to make data-driven decisions to improve customer satisfaction and loyalty.
Brands also use sentiment analysis to monitor social media platforms and news sites to gauge public opinion about their brand, marketing campaigns, or new product launches. This information can help them identify potential issues, manage public relations, and make necessary adjustments to their strategies.
Sentiment analysis plays a crucial role in social media monitoring. By analyzing the sentiment of social media posts, companies can gain valuable insights into public perception, trends, and brand sentiment. This enables them to respond promptly to customer feedback, detect potential crises, and engage with their audience effectively.
Social media sentiment analysis also provides valuable feedback for marketing campaigns. It helps businesses measure the success of their campaigns and adjust their strategies accordingly. By understanding how their audience feels about their content, businesses can improve engagement and tailor their messaging to drive better results.
Sentiment Analysis and Topic Modeling: What’s the Difference?
Summary
Sentiment analysis is like a computer trying to read our minds by analyzing text. It’s not perfect, but it can make some pretty accurate predictions about how we feel. It uses algorithms to look for clues in the words we use and the context in which we use them. However, sentiment analysis can be tricky because it can’t always understand sarcasm or the nuances of language. Overall, while sentiment analysis isn’t quite mind-reading, it can give us some insights into how people feel based on what they write.
In conclusion, sentiment analysis is a powerful tool that helps us understand people’s feelings by analyzing text. While it has its limitations, it’s still a valuable way to gain insights into public opinion and sentiment. Just remember that it’s not perfect, and sometimes it may miss important nuances in language.